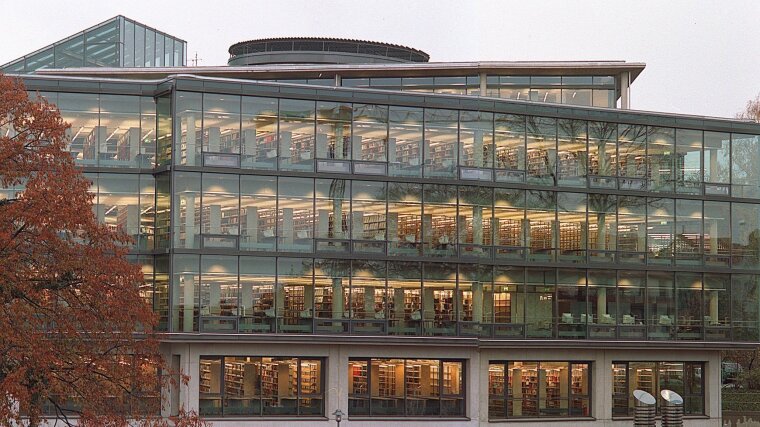
-
Influence of Occlusion in Image Classification with Self-Supervised Capsule Networks (2023)
Ladyna Wittscher, Christian Pigorsch
Occlusion significantly reduces the information content of images, corrupts object shapes and requires big data sets including a wide range of occlusion types for training. Capsule Networks are an architectural alternative to Convolutional Neural Networks for computer vision tasks that can achieve better results with more shallow networks requiring fewer parameters and generating better results for occluded images. At the same time, they suffer from issues with overfitting, slow
convergence and limited robustness. We combine a Capsule Network architecture with self-supervised learning which creates synergies, mitigates shortcomings and improves the performance up to 5.6% for MNIST with high degrees of occlusion, up to 8.2% for the combination of occlusion and data scarcity, and up to 18.1% for noise and data scarcity. Even in scenarios where the accuracy is not being improved significantly, reconstruction quality is. Self-supervision furthermore significantly reduces overfitting and does not require a huge increase in computational complexity. Additionally, we analyze the learning behaviour in detail by comparing different occlusion scenarios, pretraining different layers and parameters as well as investigating the influence of the reconstruction and margin loss on the performance.USB Proceedings of the 20th International Conference on Modeling Decisions for Artificial Intelligence, MDAI, 2023, 69–84
-
A Survey of Methods for Automated Quality Control Based on Images (2023)
Jan Diers, Christian Pigorsch
The role of quality control based on images is important in industrial production. Nevertheless, this problem has not been addressed in computer vision for a long time. In recent years, this has changed: driven by publicly available datasets, a variety of methods have been proposed for detecting anomalies and defects in workpieces. In this survey, we present more than 40 methods that promise the best results for this task. In a comprehensive benchmark, we show that more datasets and metrics are needed to move the field forward. Further, we highlight strengths and weaknesses, discuss research gaps and future research areas.
International Journal of Computer Vision, 2023, 1-29
-
Exploring Self-supervised Capsule Networks for Improved Classification with Data Scarcity (2022)
Ladyna Wittscher; Christian Pigorsch
While Capsule Networks overcome many shortcomings of Convolutional Neural Networks by taking spatial hierarchies between features into consideration and providing a new approach to routing between layers, they are sensible towards overfitting. Self-supervised training improves the semantic understanding of a network significantly and improves generalization without requiring additional images. Therefore, self-supervised Capsule Networks are a promising approach to improve learning under data scarcity, which makes the combination interesting for a huge variety of applications. Our approach improves test accuracy by up to 11.7% for small data sets and by up to 11.5% for small and imbalanced data sets. Furthermore, we explore the synergies and characteristics of this innovative method combination and give a general overview on the possibilities of self-supervised Capsule Networks.
International Conference on Image Processing and Capsule Networks, 2022, 36-50
-
Improving image classification robustness using self‐supervision (2022)
Ladyna Wittscher; Jan Diers; Christian Pigorsch
Self-supervised learning allows training of neural networks without immense, high-quality or labelled data sets. We demonstrate that self-supervision furthermore improves robustness of models using small, imbalanced or incomplete data sets which pose severe difficulties to supervised models. For small data sets, the accuracy of our approach is up to 12.5% higher using MNIST and 15.2% using Fashion-MNIST compared to random initialization. Moreover, self-supervision influences the way of learning itself, which means that in case of small or strongly imbalanced data sets, it can be prevented that classes are not or insufficiently learned. Even if input data are corrupted and large image regions are missing from the training set, self-supervision significantly improves classification accuracy (up to 7.3% for MNIST and 2.2% for Fashion-MNIST). In addition, we analyse combinations of data manipulations and seek to generate a better understanding of how pretext accuracy and downstream accuracy are related. This is not only important to ensure optimal pretraining but also for training with unlabelled data in order to find an appropriate evaluation measure. As such, we make an important contribution to learning with realistic data sets and making machine learning accessible to application areas that require expensive and difficult data collection.
Stat, 2022, Volume 11, Issue 1, e455
-
Out-of-Distribution Detection Using Outlier Detection Methods (2022)
Jan Diers; Christian Pigorsch
Out-of-distribution detection (OOD) deals with anomalous input to neural networks. In the past, specialized methods have been proposed to identify anomalous input. Similarly, it was shown that feature extraction models in combination with outlier detection algorithms are well suited to detect anomalous input. We use outlier detection algorithms to detect anomalous input as reliable as specialized methods from the field of OOD. No neural network adaptation is required; detection is based on the model’s softmax score. Our approach works unsupervised using an Isolation Forest and can be further improved by using a supervised learning method such as Gradient Boosting.
Image Analysis and Processing – ICIAP 2022, 2022, Volume 13233, 15-26
-
Self-supervised learning for outlier detection (2021)
Jan Diers; Christian Pigorsch
The identification of outliers is mainly based on unannotated data and therefore constitutes an unsupervised problem. The lack of a label leads to numerous challenges that do not occur or only occur to a lesser extent when using annotated data and supervised methods. In this paper, we focus on two of these challenges: the selection of hyperparameters and the selection of informative features. To this end, we propose a method to transform the unsupervised problem of outlier detection into a supervised problem. Benchmarking our approach against common outlier detection methods shows clear advantages of our method when many irrelevant features are present. Furthermore, the proposed approach also scores very well in the selection of hyperparameters, i.e., compared to methods with randomly selected hyperparameters.
Stat, 2021, Volume 10, Issue 1, e322
-
Approximate Bayesian Computation with Indirect Summary Statistics [submitted]
Alexander Gleim; Christian Pigorsch
Approximate Bayesian Computation (ABC) has become a popular estimation method for situations where the likelihood function of a model is unavailable. In contrast to classical Bayesian inference, this method does not build on the availability of the likelihood function, but instead relies on a distance function which measures the distance between some empirical summary statistics and their simulation based counterparts. An open question is the selection of these statistics, particularly regarding their sufficiency properties. In this paper we propose an indirect approach with summary statistics based on statistics of a suitably chosen auxiliary model. We show sufficiency of these statistics for Indirect ABC methods based on parameter estimates (ABC-IP), likelihood functions (ABC-IL) and scores (ABC-IS) of that auxiliary model. In the case of score based summary statistics we propose a weighting scheme for the different summary statistics. We illustrate the performance of these Indirect ABC methods in a simulation study and compare them to a standard ABC approach. We furthermore apply the ABC-IS method to the problem of estimating a continuous-time stochastic volatility model based on OU-processes.
[submitted]
-
Spurious Long-Range Dependence in Realized Volatilities? A Panel Examination [submitted]
Matei Demetrescu; Christian Pigorsch; Adina I. Tarcolea
Modeling volatility in a realistic manner is essential for many financial applications. One particularly relevant stylized fact of volatility proxies is their slow decay of the autocorrelation function. Although this pattern is exhibited by "real" long-memory processes as well as, spuriously, by short-memory processes with level jumps, very different strategies are implied in practice, e.g. in forecasting. Consequently, it is important to distinguish between the two sources of persistence. In this paper we propose a panel approach for analyzing the long memory property. We build on the regression based, lag-augmented version of the LM test for fractional integration and take into account all possible break points. We apply the test to investigate the long memory behavior of a high-frequency based measure of volatility of all 30 components of the Dow Jones Industrial Average between 2006 and 2008 and find that the high persistence is an artefact of mean shifts.
[submitted]
-
Estimating Residual Hedging Risk With Least-Squares Monte Carlo (2014)
Stefan Ankirchner; Christian Pigorsch; Nikolaus Schweizer
Frequently, dynamic hedging strategies minimizing risk exposure are not given in closed form, but need to be approximated numerically. This makes it difficult to estimate residual hedging risk, also called basis risk, when only imperfect hedging instruments are at hand. We propose an easy to implement and computationally efficient least-squares Monte Carlo algorithm to estimate residual hedging risk. The algorithm approximates the variance minimal hedging strategy within general diffusion models. Moreover, the algorithm produces both high-biased and low-biased estimators for the residual hedging error variance, thus providing an intrinsic criterion for the quality of the approximation. In a number of examples we show that the algorithm delivers accurate hedging error characteristics within seconds.
International Journal of Theoretical and Applied Finance, 2014, Volume 17, Issue 7
-
Beyond the Sharpe Ratio: An Application of the Aumann-Serrano Index to Performance Measurement (2012)
Ulrich Homm; Christian Pigorsch
We propose a performance measure that generalizes the Sharpe ratio. The new performance measure is monotone with respect to stochastic dominance and consistently accounts for mean, variance and higher moments of the return distribution. It is equivalent to the Sharpe ratio if returns are normally distributed. Moreover, the two performance measures are asymptotically equivalent as the underlying distributions converge to the normal distribution. We suggest a parametric and a non-parametric estimator for the new performance measure and provide an empirical illustration using mutual funds data.
Journal of Banking & Finance, 2012, Volume 36, Issue 8, 2274-2284
-
Futures Cross-hedging with a Stationary Basis (2012)
Stefan Ankirchner; Georgi Dimitroff; Gregor Heyne; Christian Pigorsch
When managing risk, frequently only imperfect hedging instruments are at hand. We show how to optimally cross-hedge risk when the spread between the hedging instrument and the risk is stationary. At the short end, the optimal hedge ratio is close to the cross-correlation of the log returns, whereas at the long end, it is optimal to fully hedge the position. For linear risk positions we derive explicit formulas for the hedge error, and for non-linear positions we show how to obtain numerically efficient estimates. Finally, we demonstrate that even in cases with no clear-cut decision concerning the stationarity of the spread it is better to allow for mean reversion of the spread rather than to neglect it.
Journal of Financial and Quantitative Analysis, 2012, Volume 47, Issue 6, 1361-1395
-
An operational interpretation and existence of the Aumann-Serrano index of riskiness (2012)
Ulrich Homm; Christian Pigorsch
In this note we provide an operational interpretation of the economic index of riskiness of Aumann and Serrano (2008) and discuss its existence in the case of non-finite gambles.
Economics Letters, 2012, Volume 114, Issue 3, 265-267
-
A Multivariate Ornstein-Uhlenbeck Type Stochastic Volatility Model [submitted]
Christian Pigorsch; Robert Stelzer
Using positive semidefinite processes of Ornstein-Uhlenbeck type a multivariate Ornstein-Uhlenbeck (OU) type stochastic volatility model is introduced. We derive many important statistical and probabilistic properties, e.g. the complete second order structure and a state-space representation. Noteworthy, many of our results are shown to be valid for the more general class of multivariate stochastic volatility models, which are driven by a stationary and square-integrable covariance matrix process. For the OU type stochastic volatility our results enable estimation and filtering of the volatility which we finally demonstrate with a short empirical illustration of our model.
[submitted]
-
Volatility estimation based on high-frequency data [forthcoming]
Uta Pigorsch; Christian Pigorsch; Ivaylo Popov
This chapter presents a review and empirical illustration of nonparametric volatility estimators that exploit the information contained in high-frequency financial data. Such ex-post volatility measures can be directly used for the modelling and forecasting of the (future) volatility dynamics, which in turn may be essential for an adequate risk management or hedging decisions. Moreover, volatility constitutes the main ingredient in asset pricing and the knowledge of this quantity therefore plays a major role in most financial applications.
forthcoming in Handbook of Computational Finance (eds. Jin-Chuan Duan, James E. Gentle and Wolfgang Härdle). New York: Springer
-
On the Definition, Stationary Distribution and Second Order Structure of Positive Semi-definite Ornstein-Uhlenbeck type Processes (2009)
Christian Pigorsch; Robert Stelzer
Several important properties of positive semi-definite processes of Ornstein-Uhlenbeck type are analysed. It is shown that linear operators of the form X→AX+XAT with A ∈ Md(ℜ) are the only ones that can be used in the definition provided one demands a natural non-degeneracy condition. Furthermore, we analyse the absolute continuity properties of the stationary distribution (especially when the driving matrix subordinator is the quadratic variation of a d-dimensional Lévy process) and study the question of how to choose the driving matrix subordinator in order to obtain a given stationary distribution. Finally, we present results on the first and second order moment structure of matrix subordinators, which is closely related to the moment structure of positive semi-definite Ornstein-Uhlenbeck type processes. The latter results are important for method of moments based estimation.
Bernoulli, 2009, Volume 15, Issue 3, 754-773
-
A Discrete-Time Model for Daily S&P500 Returns and Realized Variations: Jumps and Leverage Effects (2009)
Tim Bollerslev; George Tauchen; Christian Pigorsch; Uta Pigorsch
We develop an empirically highly accurate discrete-time daily stochastic volatility model that explicitly distinguishes between the jump and continuous-time components of price movements using nonparametric realized variation and Bipower variation measures constructed from high-frequency intraday data. The model setup allows us to directly assess the structural inter-dependencies among the shocks to returns and the two different volatility components. The model estimates suggest that the leverage effect, or asymmetry between returns and volatility, works primarily through the continuous volatility component. The excellent fit of the model makes it an ideal candidate for an easy-to-implement auxiliary model in the context of indirect estimation of empirically more realistic continuous-time jump diffusion and Lévy-driven stochastic volatility models, effectively incorporating the interdaily dependencies inherent in the high-frequency intraday data.
Journal of Econometrics, 2009, Volume 150, Issue 2, 151-166
-
Financial Economics, Fat-tailed Distributions
Markus Haas; Christian Pigorsch
This article reviews some of the most important concepts and distributional models that are used in empirical finance to capture the (almost) ubiquitous stochastic properties of returns.
in Encyclopedia of Complexity and Systems Science (ed. Robert A. Meyers), pp. 3404-3435. New York: Springer
-
The Volatility of Realized Volatility (2008)
Fulvio Corsi; Stefan Mittnik; Christian Pigorsch; Uta Pigorsch
In recent years, with the availability of high-frequency financial market data modeling realized volatility has become a new and innovative research direction. The construction of observable or realized volatility series from intra-day transaction data and the use of standard time-series techniques has lead to promising strategies for modeling and predicting (daily) volatility. In this article, we show that the residuals of commonly used time-series models for realized volatility and logarithmic realized variance exhibit non-Gaussianity and volatility clustering. We propose extensions to explicitly account for these properties and assess their relevance for modeling and forecasting realized volatility. In an empirical application for S&P500 index futures we show that allowing for time-varying volatility of realized volatility and logarithmic realized variance substantially improves the fit as well as predictive performance. Furthermore, the distributional assumption for residuals plays a crucial role in density forecasting.
Econometric Reviews, 2008, Volume 27, Issue 1-3, 46-78